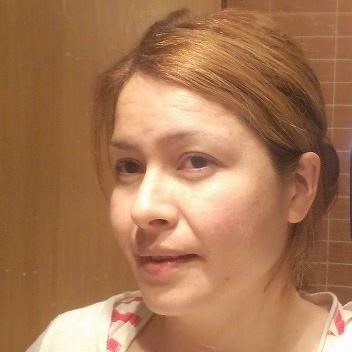
Betsabé Perez Garrido
Betsabé Perez Garrido holds a PhD in Mathematical Engineering (specializing in Statistics) from the Universidad Carlos III de Madrid (Spain). Her research interests focus on: lineal mixed models outlier detection and applied statistics. In 2014 she won the best paper award in the MakeLearn (Management, Learning and Knowledge) International Conference in Portoroz, Slovenia. Her recent publications are:
Pérez, B., Molina, I., Thieler, A., Fried, R. and Peña, D. (2017). “Fast and robust estimators of variance components in the nested error model”. Statistics and Computing, (accepted to appear)
Sebrek, Sz., and Pérez, B. (2015). “Competitive pressure and technological degree of the product portfolio: Implications for firm performance”. Acta Oeconomica, 65(2), 211-229.
Pérez, B., Molina, I., & Peña, D. (2014). “Outlier detection and robust estimation in linear regression models with fixed group effects”. Journal of Statistical Computation and Simulation, 84(12), 2652-2669.
Research topic
Large-scale datasets generally cover a large and considerable portion of the population within a territory, often reaching nationwide and even worldwide coverage. Today, most of the current statistical methods are appropriate for the creation of global indicators. However in practice, government agencies or policymakers also have special interest in the creation of local indicators and particularly in regions where the number of available information is too small (called small areas). Small area estimation (SAE) is a special branch of statistics which is appropriate for the creation of local indicators within small areas. The SAE approach uses extensively linear mixed models. A major problem arises when there are outliers in the data since they have the potential to destroy the estimation of local indicators. Thus, the aim of this research is to explore a new statistical methodology for the creation of local indicators under the presence of outlier observations. The new methodology will be illustrated using a real dataset.